Identifying Data Set for Dissertation
Abstract:
The essential step in authorizing a proposed system is to evaluate over an appropriate dataset. The value that has to be generated from data needs the ability to find out, access, and give sense to datasets. Many efforts are carried out to encourage data sharing and reuse of data, from publishers asking writers to submit data along with dissertation to open data portals, data marketplaces, and data communities. Google recently released a service for identifying datasets, which lets users find out data that has been stored in different online repositories through keyword queries. These developments predict a research field that has been emerging in identifying dataset or data retrieval that broadly contains frameworks, tools, and methods that helps in comparing a user data need over a collection of datasets. 1
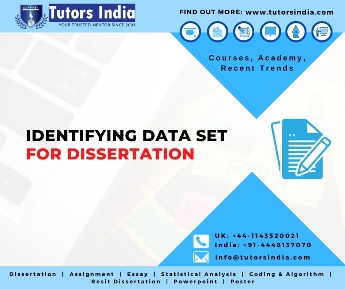
Introduction
The process of collecting and validating data on variables of interest is data set collection. It enables us to answer research questions, analyze hypotheses, and assess the outcome. Data collection is one of the significant processes in conducting research. It is good to have the best research design in the world but if it is not possible to collect the required data then completing the dissertation becomes a difficult task. Data collection is a very challenging task that requires systematic planning, patience, hard work, perseverance, and more to complete the project successfully. 2
Categories of Data Set
Data are classified into two major categories: qualitative and quantitative.
i. Qualitative Data: Qualitative data are usually descriptive and non-numerical in nature. The qualitative data collection method plays a significant role in impact evaluation by providing data that can be helpful to understand the processes behind observed outcomes. Moreover, these methods can also improve the quality of survey-based quantitative evaluations which helps to generate evaluation hypotheses and strengthens the design of survey questionnaires. It also helps in expanding or clarifying findings of quantitative evaluation.
ii. Quantitative Data: Quantitative data is numerical and can be computed mathematically. This method uses various scales, such as ordinal scale, nominal scale, ratio scale, and interval scale. These approaches are very cheaper to implement, can be easily compared as they are standardized. However, these approaches are limited in their ability for the research and clarification of similarities and differences. The outcome obtained from these methods can be summarized, compared, and generalized very easily. 3
Types of Data Set Searches

Source: https://link.springer.com/article/10.1007/s00778-019-00564-x
1. Hidden search: The hidden or deep search refers to the content that can be found behind web forms usually written in HTML. There are two main approaches for finding data on the deep web. The first is the traditional method to develop vertical search engines, where semantic mappings are created between each website and a centralized third party customized to a specific domain. Structured queries are created on the third party and redirected through the web forms using mappings. A second approach tries to produce the resulting web pages in HTML that emerge from web form searches. Google has proposed an approach for finding data in deep web content by approximating input automatically to quite a few million HTML forms that are written in multiple languages and span over hundreds of domains, and the resulting HTML pages are added to its search engine index. The user will be directed to the result of the newly submitted form when they click on a search result.
2. Entity-centric search: In this type of search, information is ordered and accessed through entities of interest, and their relationships and attributes.
3. Tabular search: In tabular search, users access the data stored in one or more tables. The main aim is to identify specific data, such as attribute names or extending tables with fresh attributes. 4
Decentralized Data Set Search
4. Google Dataset Search: Google proposed a vertical search engine that has been created to identify datasets on the web. This system utilizes schema.org and DCAT. The web for all datasets are crawled based on Google web crawl with the use of the schema.org, as well as the datasets that are described using DCAT, and gathers the associated metadata. They additionally link the metadata to some other sources, find out a replica and generate an index of enhanced metadata for each dataset. The metadata is submissive to the knowledge graph of Google and its search capabilities that are built on the top of this metadata. The datasets that are indexed can be identified through keywords and CQL expressions.
5. Domain-specific search: In this type of search, services focus on datasets from specific domains. They propose modified metadata schemas to explain the datasets and crawlers are implemented to determine them automatically. 5
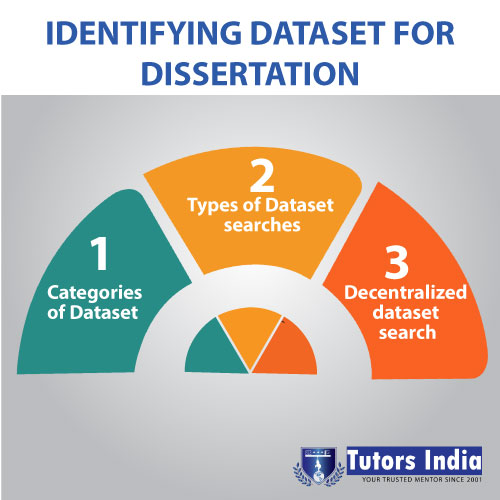
Some recent topics in dataset:
- Dimensional Reduction approaches for large scale data.
- Training / Inference in noisy environments and incomplete data.
- Handling uncertainty in big data processing.
- Anomaly Detection in Very Large Scale Systems.
- Scalable privacy preservation on big data.
- Lightweight Big Data analytics as a Service.
- Approaches to make the models learn with less number of data samples.
Conclusion
There are several ways to widen the system’s functionality. Academic search engines extract metadata, in the form of a list of authors, publication year, venue, and so on. The first step will be to provide this data for each paper in the system. But apart from this, it will be useful to apply this information to datasets, to find out the authors and venues that have used a dataset, and also to determine how its usage has changed over time. Users of the system also help in enhancing the quality of search, by giving feedback on the extracted links, signifying errors, and finding out datasets within papers that cannot be identified by the classifier. With such participation, the system’s accuracy, recall, and coverage can be enhanced further. Several future improvements must be carried out to get better results. One of the ways is to study the global data collection patterns after this system is fully organized and employed by many users in real-life situations. This can be achieved mainly by applying machine learning algorithms such as clustering algorithms like k-Means Clustering to split the users into several clusters. 6
References:
- Melissa P. Johnston, 2019, Secondary Data Analysis: A Method of which the Time Has Come
- Adriane Chapman, Elena Simperl, Laura Koesten, George Konstantinidis, Luis-Daniel Ibáñez, Emilia Kacprzak, Paul Groth, 2020, Dataset search: a survey
- Syed Muhammad Sajjad Kabir, 2020, Methods of Data Collection.
- Meiyu Lu, Srinivas Bangalore, Graham Cormode, Marios Hadjieleftheriou, Divesh Srivastava, A Dataset Search Engine for the Research Document Corpus
- Chinelo Igwenagu, 2021, Fundamentals of Research Methodology and Data Collection
- Sage pub., 2021, How to Complete Dissertation Using Online Data Access and Collection.