Business Analytics Dissertation Help: Defining And Identifying A Research Agenda For An Evidence Based Management Framework
In Brief (Highlights)
- You will find the best dissertation research areas/topics for future researchers enrolled in Engineering and Technology.
- In order to identify future research topics, we have reviewed the technical conventions. (recent peer-reviewed studies).
- Business analytics refers to the systematic use of data collected from a variety of sources, quantitative and statistical analysis, predictive and explanatory models and evidence-based management to direct actions and decisions towards the appropriate stakeholders.
- Writing a business analytics dissertations, it is important for the researchers to have strong knowledge related to the approaches used and in the data science and machine learning which will help in writing business analytics dissertation.
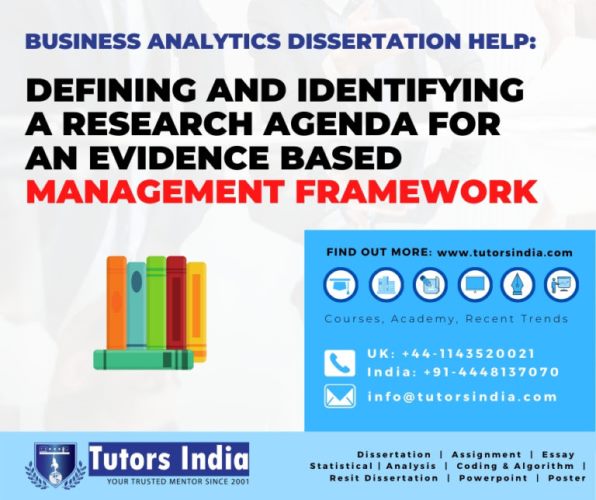
Organizations employ business analytics to make intelligent decisions, which can be made quicker and better in order to enhance the value of the business. Until today, academicians and industrialists have focused mainly on descriptive and predictive analytics. However, prescriptive analytics is gaining huge interest in terms of research in the business analytics area as it is considered as the best course of action for future businesses. Therefore Prescriptive analytics is often considered as the next step in improving data analytics maturity, which further leads to augmented decision making improving business performance.
You will find the best Dissertation writing for future researchers enrolled in Business and Management. These topics are researched in-depth at the University of Glasgow, UK, Sun Yat-sen University, University of St Andrews and many more.
Business analytics refers to the systematic
Download our Dissertation writing related Reference book papers such as tutorials, proprietary materials, research projects and many more @ tutorsindia.com/academy/books
Therefore, while writing a business analytics dissertations, it is important for the researchers to have strong knowledge related to the approaches used and in the data science and machine learning which will help in writing business analytics dissertation.
For the research agenda and creating an evidence-based management framework
Association to Advance Collegiate Schools of Business (AACSB), 2018 states that “In today’s proliferating dynamic business environment, business schools must react to the business world’s changing needs by providing relevant knowledge and skills to the communities they serve” (Association to Advance Collegiate Schools of Business (AACSB), 2018). An Evidence-Based Management (EBMgt) methodology addresses highly important business issues using effective methodologies and implementations (Pfeffer & Sutton, 2006). During the last two decades, the prevalence of EBMgt in research has increased. It was put forward as a way of bridging the gap between research and practice, EBMgt is a data and theory-driven approach to decision-making, arguing that while managers cannot have full knowledge in ever-changing environments, the consistency of decisions is usually enhanced by taking data-driven facts into consideration (Pfeffer & Sutton, 2006; Pfeffer, 2012).
Tutors India has vast experience in writing Dissertation for Business and Management students pursuing in UK. Order Now
It is “about making decisions by the diligent, clear, and judicious use of four sources of knowledge: The classical concept of decision support systems (DSS) is a computer-based information systemleveraging data and/or models to support decision-makers solve semi-structured or unstructured problems (Turban, Aronson, & Liang, 2004). The classical what-if analysis of DSS is to take a question, apply a data-driven model to evaluate an outcome and compare that outcome with alternative interventions. This concept of data-driven decision making is an integral part of the Business Analytics System (BAF) of Holsapple, Lee-Post and Pakath, in which evidence-based problem identification and solutions occur in a business context (Holsapple, Lee-Post, & Pakath, 2014). Decisions powered by data are evidence-based and companies step into evidence-based analytics to obtain a competitive advantage (Cho, Song, Comuzzi, & Yoo, 2017; Davenport, 2006; Wimmer, Yoon, & Sugumaran, 2016). DSS as a business analytics program, therefore, belongs to EBMgt’s assessed by external evidence circle in Figure 1 (Scheibe, Nilakanta, Ragsdale, & Younie, 2019)
In order to identify the sources for technical writing, we have reviewed the Dissertation document (recent peer-reviewed topics) on Business and Management.
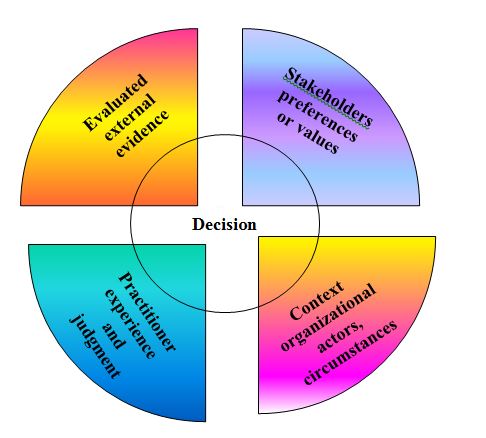
The intersection of EBMgt and analytics is especially important for research on management science but is often only discussed in passing. In addition, this framework highlights the importance of data, models, stakeholders and context in developing and implementing business analytics solutions through the four elements of EBMgt. Companies use analytics to succeed through advances in automated business processes and are enabled by predictive analytics (Prahalad & Krishnan, 1999).
DSS research has lasted for almost half a century, but interest in DSS techniques, methods, and implementation has recently increased under the umbrella of “big data” and “business analytics” (Holsapple et al., 2014). Therefore, EBMgt is concerned with making decisions by using conscientiously, specifically and judiciously the best available data from various sources to maximize the likelihood of favourable outcomes. This can be accomplished through the six A’s: (1) Asking: transforming a real-world problem into an answerable question. (2) Acquiring: the systematic search and gathering of proof. (3) Appraising: to objectively evaluate the quality and validity of the evidence. (4) Aggregating: weighing proof and putting it all together. (5) Applying: Inclusion of evidence in the process of decision-making. (6) Assessing: Evaluation of the outcomes of the decision adopted. The intersection of EBMgt and analytics is particularly important for research in management sciences.
Therefore, writing a dissertationin the field of business analytics can be challenging; however, it is important to have a thorough understanding of the business analytics and evidence-based management and their correlation.
References
- Cho, M., Song, M., Comuzzi, M., & Yoo, S. (2017). Evaluating the effect of best practices for business process redesign: An evidence-based approach based on process mining techniques. Decision Support Systems, 104, 92–103. https://doi.org/10.1016/j.dss.2017.10.004
- Davenport, T. H. (2006). Competing on analytics. Harvard Business Review, 84(1), 98. Retrieved from http://www.impactline.net/%C0%DA%B7%E1%C3%B7%BA%CE%B9%B0/OLAPDW/AnalyticsHBR.pdf
- Davenport, T. H., & Harris, J. G. (2007). Competing on Analytics. The New Science of Winning.�Harvard Business School Press, Boston, MA., 2007. Google Scholar Google Scholar Digital Library Digital Library. Retrieved from https://lib.ugent.be/en/catalog/rug01:001210069
- Holsapple, C., Lee-Post, A., & Pakath, R. (2014). A unified foundation for business analytics. Decision Support Systems, 64, 130–141. https://doi.org/10.1016/j.dss.2014.05.013
- Krumeich, Christ, M., Julian, & Kempa-Liehr, A. W. (2016). Integrating Predictive Analytics into Complex Event Processing by Using Conditional Density Estimations. 2016 IEEE 20th International Enterprise Distributed Object Computing Workshop (EDOCW), 1–8. https://doi.org/10.1109/EDOCW.2016.7584363
- Lepenioti, K., Bousdekis, A., Apostolou, D., & Mentzas, G. (2020). Prescriptive analytics: Literature review and research challenges. International Journal of Information Management, 50, 57–70. https://doi.org/10.1016/j.ijinfomgt.2019.04.003
- Mikalef, P., Pappas, I. O., Krogstie, J., & Giannakos, M. (2018). Big data analytics capabilities: a systematic literature review and research agenda. Information Systems and E-Business Management, 16(3), 547–578. https://doi.org/10.1007/s10257-017-0362-y
- Mortenson, M. J., Doherty, N. F., & Robinson, S. (2015). Operational research from Taylorism to Terabytes: A research agenda for the analytics age. European Journal of Operational Research, 241(3), 583–595. https://doi.org/10.1016/j.ejor.2014.08.029
- Pfeffer, J. (2012). Evidence-based management for entrepreneurial environments: Faster and better decisions with less risk. In Chance and Intent (pp. 71–82). Retrieved from https://www.taylorfrancis.com/books/e/9780203126677/chapters/10.4324/9780203126677-11
- Pfeffer, J., & Sutton, R. I. (2006). Management half-truths and nonsense: How to practice evidence-based management. California Management Review, 48(3), 77–100. Retrieved from https://journals.sagepub.com/doi/pdf/10.1177/000812560604800301
- Prahalad, C. K., & Krishnan, M. S. (1999). The new meaning of quality in the information age. Harvard Business Review, 77(5), 109. Retrieved from https://go.gale.com/ps/i.do?id=GALE%7CA55739484&sid=googleScholar&v=2.1&it=r&linkaccess=abs&issn=00178012&p=AONE&sw=w
- Scheibe, K. P., Nilakanta, S., Ragsdale, C. T., & Younie, B. (2019). An evidence-based management framework for business analytics. Journal of Business Analytics, 2(1), 47–62. https://doi.org/10.1080/2573234X.2019.1609341
- Soltanpoor, R., & Sellis, T. (2016). Prescriptive analytics for big data. Australasian Database Conference, 245–256. Retrieved from https://link.springer.com/chapter/10.1007/978-3-319-46922-5_19
- Turban, E., Aronson, J. E., & Liang, T.-P. (2004). No Title. Decision Support Systems and Intelligent Systems. Retrieved from https://dl.acm.org/doi/book/10.5555/994103
- Vidgen, R., Shaw, S., & Grant, D. B. (2017). Management challenges in creating value from business analytics. European Journal of Operational Research, 261(2), 626–639. Retrieved from https://www.sciencedirect.com/science/article/pii/S0377221717301455
- Wimmer, H., Yoon, V. Y., & Sugumaran, V. (2016). A multi-agent system to support evidence based medicine and clinical decision making via data sharing and data privacy. Decision Support Systems, 88, 51–66. https://doi.org/10.1016/j.dss.2016.05.008